How does study-abroad impact the development of grammatical accuracy? An Interview with Dr. Hang Du
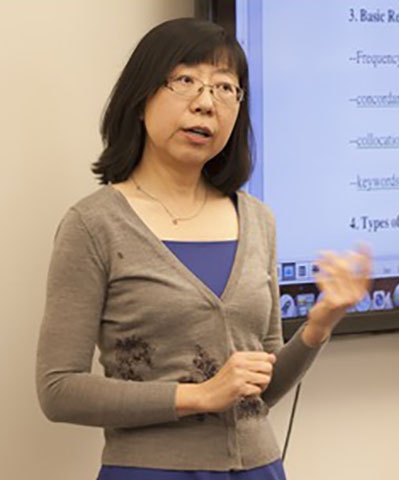
Dr. Hang Du of Middlebury’s Chinese Department has for over a decade been building and analyzing a database of spoken Chinese to understand how students studying abroad learn Chinese. We checked in with her to see how she is doing this work, what she has learned, and what advice she has for others embarking on this type of study.
Tell me a bit about your current project. What is the question you are asking? How are you going about answering this question?
The questions that I’m asking are “Did students make progress in their Chinese proficiency as a result of study abroad in China? If so, what kind of progress did they make (in the areas of grammar, vocabulary, etc.)?” I’m trying to answer these questions by analyzing data in a corpus of over a million Chinese characters of transcribed student speech—the result of my study abroad research that I did for almost ten years.
Where do you get your data? What is the source for the transcribed speech?
Between 2006 and 2014, I conducted research with students who spent one semester or an academic year studying in our program in China. A total number of 83 students (39 males and 44 females) participated in the study. Data were individual conversations in Chinese between me and the participating students in person or via Skype about once a month, resulting in four conversations for each semester student and seven for each year long student. The conversations were semi-structured. Students were encouraged to talk about anything that they wanted to talk about, but when they ran out of things to say, I had some questions. The main purpose was to encourage the students to talk as freely as possible without feeling any stress. Each conversation was about 30 minutes long and was recorded with a digital device. Each recording was later transcribed with simplified Chinese characters into a Microsoft Word document, which was later converted to a plain text document as one file using the UTF-8 encoding system. In the process my lines and non-Chinese characters such as Arabic numerals and roman letters were removed, leaving only student data in Chinese characters, resulting in 1,018,171 characters in the corpus.
How did you analyze the data that you collected? What tools and methods did you use?
Some basic methods used in corpus linguistics research including concordancing, also known as “keyword in context” (KWIC), frequency lists, and keyword analyses were used with AntConc, which has built-in statistical analysis tools. The investigation went in the following three different, yet related directions: 1) keyword analyses between “before” and “after” study abroad. Keywords are words that are significantly more frequent in one corpus than in another corpus. I used this method to compare all the data from the first conversation and all the data from the last conversation to see how the students used words differently in the two conversations to see if they made progress in their grammar and vocabulary use. 2) the use of the Chinese perfective aspect marker -le, which previous research revealed that it’s very difficult for second language learners to fully acquire. I used Antconc to extract all the instances of this word in the “before” and “after” corpora. After that the analyses had to be done manually to determine whether the word was used correctly or not. After that, statistical tests were used with the statistical packages SPSS and SAS to determine if the differences were statistically significant. 3) The keyword analyses from 1) above suggested that the students’ use of the Chinese word for “but” moved towards the native norm at the end of study in China. In order to confirm that, a list of free online native Chinese corpora and a corpus of native spoken Chinese from the Linguistic Data Consortium ( https://www.ldc.upenn.edu/) were consulted.
What did you discover?
Results show that after study abroad in China, the top ten words that the students used significantly more than before are in general more sophisticated, suggesting progress in their lexical development; they made significant progress in the accuracy of using the perfective aspect marker-le; and for the two Chinese words that mean “but” in English, they shifted from using kěshì, which is less frequent in native use, to the more frequent dànshì, suggesting moving towards the native norm.
Do you have plans for a next phase of this project?
The manuscript that reported the results of this research is undergoing peer review for a journal right now. In the next stage of this research, the acquisition of other aspects of Chinese grammar will be studied. Additionally, since data were collected once a month during the study abroad period, data from different stages of that period will be compared to see if students made progress from the first month, to the second month, to the third month, and so on. It would also be interesting to analyze the semester and yearlong students’ data for similarities and differences in terms of their acquisition of various aspects of the language. Methodologically, other methods and techniques commonly used in corpus linguistics research, such as collocations (words that often occur together), will be utilized. I would eventually like to make this corpus freely available online for other researchers world-wide to use. In order to achieve that goal, more work needs to be done in the corpus construction, such as adding more metadata, further removing any information that could potentially identify the students, and providing error tagging.
As you think about this project, what have you learned in general about this sort of data intensive research? And what advice might you have for others that are considering pursuing this type of inquiry?
I started building the corpus during my 2014-2015 sabbatical by transcribing the recordings. It was a long process. Even though there are many large corpora in many languages out there, including learner corpora, sizable spoken corpora are still relatively rare, because automatic machine transcription of any language is still far less accurate than transcription done by humans, and transcribing learner speech is even trickier due to learners’ non-standard pronunciation, and unusual collocations. So the first thing that I learned is, even though this project is trail-brazing and exciting, the work was daunting. Be prepared. Once the recordings were transcribed, I relied on people who were proficient in the computer program Python to help me clean the data by removing unwanted elements, such as my lines, roman letters, etc. I would like to take a course to learn a basic computer programming language such as Python or R during this sabbatical, but it didn’t happen due to the pandemic. It would be great if I had such skills myself. The last thing that I learned was that even though computer programs can do some basic analyses such as word frequency, keyword comparison, etc., some analyses, such as counting grammatical errors, had to be done manually. For example, for the use of the Chinese perfective marker -le, I was able to extract all the instances of the word with AntConc, but after that I had to manually examine each line (about 3,000 of them) to determine if each -le was used correctly or not in the context. However, for underuse (places where -le should have been used but was not), I had to go back to the original transcriptions in Word documents, which include what I said in those conversations for the full context, to find all the instances of underuse.
To summarize: 1) Be prepared for the amount of work involved if one wants to build a spoken learner corpus like mine. 2) If possible, learn the basics of a computer programming language such as Python or R, or better, work with a collaborator who has expertise in this area. 3) Unless one is working with a corpus that is already fully POS (part-of-speech) tagged and error-tagged, there’s still a lot of analyses that have to be done manually. Existing error-tagged spoken learner corpora are hard to find, especially in language other than ESL, but even if one could find such a corpus, if the errors that are already marked are not what the research is interesting in, then they would have to do their own manually analyses. But I think this is okay because after all, this kind of research is still in the humanities, so a little “human touch” is acceptable.