Alex Lyford
Assistant Professor of Mathematics and Statistics, Associate Director of the Middlebury Initiative for Data and Digital Methods
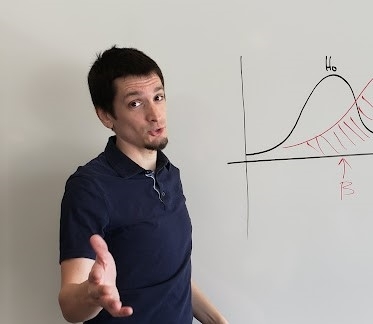
- Office
- Warner 210
- Tel
- (802) 443-5564
- alyford@middlebury.edu
- Office Hours
- Spring 2025: Tuesday 11--12, Wednesday 3--Infinity, Thursday 11--12, and by appointment.
Alex Lyford is an Assistant Professor of Statistics, and he has been at Middlebury College since 2017. He received a Ph.D. in Statistics from the University of Georgia, and his research areas of interest are machine learning, text analysis, statistics education, and math games. Alex’s hobbies include sports, hiking, and playing board games. Alex also hosts Board Game Night in the Math department once a month on Mondays.
Students interested in doing research with Alex should stop by his office any time or contact him via email.
Courses Taught
BIOL 1230
DataScience Across Disciplines
Course Description
Data Science Across Disciplines
In this course, we will gain exposure to the entire data science pipeline—obtaining and cleaning, large and messy data sets, exploring these data and creating engaging visualizations, and communicating insights from the data in a meaningful manner. During morning sessions, we will learn the tools and techniques required to explore new and exciting data sets. During afternoon sessions, students will work in small groups with one of several faculty members on domain-specific research projects in Biology, Geography, History, Mathematics/Statistics and Sociology. This course will use the R programming language. No prior experience with R is necessary.
BIOL 1230: Students enrolled in Professor Casey’s (Biology) afternoon section will use the tools of data science to investigate the drivers of primary production in the Southern Ocean around Antarctica. To do this, students will learn to manipulate, interrogate, and visualize global ocean satellite datasets.
GEOG 1230: In this section, we will investigate human vulnerability to natural hazards in the United States using location-based text data about hurricane and flood disasters from social media. We will analyze data qualitatively, temporally, and spatially to gain insights into the human experience of previous disasters and disaster response. We will present findings using spatial data visualizations with the aim of informing future disaster preparedness and resilience.
HIST 1230: In U.S. history, racial differences and discrimination have powerfully shaped who benefited from land and farm ownership. How can historians use data to understand the history of race and farming? Students will wrangle county- and state-level data from the U.S. Census of Agriculture from 1840-1912 to create visualizations and apps that allow us to find patterns in the history of race and land, to discover new questions we might not know to ask, and to create tools to better reveal connections between race, land, and farming for a general audience.
STAT 1230: In this course students will dive into the world of data science by focusing on invasive species monitoring data. Early detection is crucial to controlling many invasive species; however, there is a knowledge gap regarding the sampling effort needed to detect the invader early. In this course, we will work with decades of invasive species monitoring data collected across the United States to better understand how environmental variables play a role in the sampling effort required to detect invasive species. Students will gain experience in the entire data science pipeline, but the primary focus will be on data scraping, data visualization, and communication of data-based results to scientists and policymakers.
SOCI 1230: Do sports fans care about climate change? Can sports communication be used to engage audiences on environmental sustainability? In this section of the course, students will use the tools of data science to examine whether interest in sports is associated with climate change knowledge, attitudes and behaviors, as well as other political opinions. Participants will use survey data to produce visualizations and exploratory analyses about the relationship between sports fandom and attitudes about environmental sustainability.
Terms Taught
Requirements
ENVS 1230
DataScience Across Disciplines
Course Description
Data Science Across Disciplines
In this course, we will gain exposure to the entire data science pipeline—obtaining and cleaning large and messy data sets, exploring these data and creating engaging visualizations, and communicating insights from the data in a meaningful manner. During morning sessions, we will learn the tools and techniques required to explore new and exciting data sets. During afternoon sessions, students will work in small groups with one of several faculty members on domain-specific research projects in Sociology, Neuroscience, Animation, Art History, or Environmental Science. This course will utilize the R programming language. No prior experience with R is necessary.
ENVS: Students will engage in research within environmental health science—the study of reciprocal relationships between human health and the environment. High-quality data and the skills to make sense of these data are key to studying environmental health across diverse spatial scales, from individual cells through human populations. In this course, we will explore common types of data and analytical tools used to answer environmental health questions and inform policy.
FMMC: Students will explore how to make a series of consequential decisions about how to present data and how to make it clear, impactful, emotional or compelling. In this hands-on course we will use a wide range of new and old art making materials to craft artistic visual representations of data that educate, entertain, and persuade an audience with the fundamentals of data science as our starting point.
NSCI/MATH: Students will use the tools of data science to explore quantitative approaches to understanding and visualizing neural data. The types of neural data that we will study consists of electrical activity (voltage and/or spike trains) measured from individual neurons and can be used to understand how neurons respond to and process different stimuli (e.g., visual or auditory cues). Specifically, we will use this neural data from several regions of the brain to make predictions about neuron connectivity and information flow within and across brain regions.
SOCI: Students will use the tools of data science to examine how experiences in college are associated with social and economic mobility after college. Participants will combine sources of "big data" with survey research to produce visualizations and exploratory analyses that consider the importance of higher education for shaping life chances.
HARC: Students will use the tools of data science to create interactive visualizations of the Dutch textile trade in the early eighteenth century. These visualizations will enable users to make connections between global trade patterns and representations of textiles in paintings, prints, and drawings.
Terms Taught
Requirements
FMMC 1230
DataScience Across Disciplines
Course Description
Data Science Across Disciplines
In this course, we will gain exposure to the entire data science pipeline—obtaining and cleaning large and messy data sets, exploring these data and creating engaging visualizations, and communicating insights from the data in a meaningful manner. During morning sessions, we will learn the tools and techniques required to explore new and exciting data sets. During afternoon sessions, students will work in small groups with one of several faculty members on domain-specific research projects in Sociology, Neuroscience, Animation, Art History, or Environmental Science. This course will utilize the R programming language. No prior experience with R is necessary.
ENVS: Students will engage in research within environmental health science—the study of reciprocal relationships between human health and the environment. High-quality data and the skills to make sense of these data are key to studying environmental health across diverse spatial scales, from individual cells through human populations. In this course, we will explore common types of data and analytical tools used to answer environmental health questions and inform policy.
FMMC: Students will explore how to make a series of consequential decisions about how to present data and how to make it clear, impactful, emotional or compelling. In this hands-on course we will use a wide range of new and old art making materials to craft artistic visual representations of data that educate, entertain, and persuade an audience with the fundamentals of data science as our starting point.
NSCI/MATH: Students will use the tools of data science to explore quantitative approaches to understanding and visualizing neural data. The types of neural data that we will study consists of electrical activity (voltage and/or spike trains) measured from individual neurons and can be used to understand how neurons respond to and process different stimuli (e.g., visual or auditory cues). Specifically, we will use this neural data from several regions of the brain to make predictions about neuron connectivity and information flow within and across brain regions.
SOCI: Students will use the tools of data science to examine how experiences in college are associated with social and economic mobility after college. Participants will combine sources of "big data" with survey research to produce visualizations and exploratory analyses that consider the importance of higher education for shaping life chances.
HARC: Students will use the tools of data science to create interactive visualizations of the Dutch textile trade in the early eighteenth century. These visualizations will enable users to make connections between global trade patterns and representations of textiles in paintings, prints, and drawings.
Terms Taught
Requirements
FYSE 1216
Upcoming
Mathematics of Board Games
Course Description
Mathematics of Board Games
People have been playing games since as early as 2000 B.C. Since then, avid players have devised strategies to maximize their chances of winning. In this course we will dissect a variety of modern board games and analyze various strategies for each game using mathematics, computers, and intuition. We will further discuss whether an optimal strategy exists for each game and propose modifications to existing rules and scoring schemes. The course will culminate with a project to construct a board game. All are welcome regardless of mathematical background. 3 hrs. sem
Terms Taught
Requirements
GEOG 1230
DataScience Across Disciplines
Course Description
Data Science Across Disciplines
In this course, we will gain exposure to the entire data science pipeline—obtaining and cleaning, large and messy data sets, exploring these data and creating engaging visualizations, and communicating insights from the data in a meaningful manner. During morning sessions, we will learn the tools and techniques required to explore new and exciting data sets. During afternoon sessions, students will work in small groups with one of several faculty members on domain-specific research projects in Biology, Geography, History, Mathematics/Statistics and Sociology. This course will use the R programming language. No prior experience with R is necessary.
BIOL 1230: Students enrolled in Professor Casey’s (Biology) afternoon section will use the tools of data science to investigate the drivers of tick abundance and tick-borne disease risk. To do this students will draw from a nation-wide ecological database.
GEOG 1230: In this section, we will investigate human vulnerability to natural hazards in the United States using location-based text data about hurricane and flood disasters from social media. We will analyze data qualitatively, temporally, and spatially to gain insights into the human experience of previous disasters and disaster response. We will present findings using spatial data visualizations with the aim of informing future disaster preparedness and resilience.
HIST 1230: In U.S. history, racial differences and discrimination have powerfully shaped who benefited from land and farm ownership. How can historians use data to understand the history of race and farming? Students will wrangle county- and state-level data from the U.S. Census of Agriculture from 1840-1912 to create visualizations and apps that allow us to find patterns in the history of race and land, to discover new questions we might not know to ask, and to create tools to better reveal connections between race, land, and farming for a general audience.
STAT 1230: In this course students will dive into the world of data science by focusing on invasive species monitoring data. Early detection is crucial to controlling many invasive species; however, there is a knowledge gap regarding the sampling effort needed to detect the invader early. In this course, we will work with decades of invasive species monitoring data collected across the United States to better understand how environmental variables play a role in the sampling effort required to detect invasive species. Students will gain experience in the entire data science pipeline, but the primary focus will be on data scraping, data visualization, and communication of data-based results to scientists and policymakers.
SOCI 1230: Do sports fans care about climate change? Can sports communication be used to engage audiences on environmental sustainability? In this section of the course, students will use the tools of data science to examine whether interest in sports is associated with climate change knowledge, attitudes and behaviors, as well as other political opinions. Participants will use survey data to produce visualizations and exploratory analyses about the relationship between sports fandom and attitudes about environmental sustainability.
Terms Taught
Requirements
HARC 1230
DataScience Across Disciplines
Course Description
Data Science Across Disciplines
In this course, we will gain exposure to the entire data science pipeline—obtaining and cleaning large and messy data sets, exploring these data and creating engaging visualizations, and communicating insights from the data in a meaningful manner. During morning sessions, we will learn the tools and techniques required to explore new and exciting data sets. During afternoon sessions, students will work in small groups with one of several faculty members on domain-specific research projects in Sociology, Neuroscience, Animation, Art History, or Environmental Science. This course will utilize the R programming language. No prior experience with R is necessary.
ENVS: Students will engage in research within environmental health science—the study of reciprocal relationships between human health and the environment. High-quality data and the skills to make sense of these data are key to studying environmental health across diverse spatial scales, from individual cells through human populations. In this course, we will explore common types of data and analytical tools used to answer environmental health questions and inform policy.
FMMC: Students will explore how to make a series of consequential decisions about how to present data and how to make it clear, impactful, emotional or compelling. In this hands-on course we will use a wide range of new and old art making materials to craft artistic visual representations of data that educate, entertain, and persuade an audience with the fundamentals of data science as our starting point.
NSCI/MATH: Students will use the tools of data science to explore quantitative approaches to understanding and visualizing neural data. The types of neural data that we will study consists of electrical activity (voltage and/or spike trains) measured from individual neurons and can be used to understand how neurons respond to and process different stimuli (e.g., visual or auditory cues). Specifically, we will use this neural data from several regions of the brain to make predictions about neuron connectivity and information flow within and across brain regions.
SOCI: Students will use the tools of data science to examine how experiences in college are associated with social and economic mobility after college. Participants will combine sources of "big data" with survey research to produce visualizations and exploratory analyses that consider the importance of higher education for shaping life chances.
HARC: Students will use the tools of data science to create interactive visualizations of the Dutch textile trade in the early eighteenth century. These visualizations will enable users to make connections between global trade patterns and representations of textiles in paintings, prints, and drawings.
Terms Taught
Requirements
HIST 1230
DataScience Across Disciplines
Course Description
Data Science Across Disciplines
In this course, we will gain exposure to the entire data science pipeline—obtaining and cleaning, large and messy data sets, exploring these data and creating engaging visualizations, and communicating insights from the data in a meaningful manner. During morning sessions, we will learn the tools and techniques required to explore new and exciting data sets. During afternoon sessions, students will work in small groups with one of several faculty members on domain-specific research projects in Biology, Geography, History, Mathematics/Statistics and Sociology. This course will use the R programming language. No prior experience with R is necessary.
BIOL 1230: Students enrolled in Professor Casey’s (Biology) afternoon section will use the tools of data science to investigate the drivers of tick abundance and tick-borne disease risk. To do this students will draw from a nation-wide ecological database.
GEOG 1230: In this section, we will investigate human vulnerability to natural hazards in the United States using location-based text data about hurricane and flood disasters from social media. We will analyze data qualitatively, temporally, and spatially to gain insights into the human experience of previous disasters and disaster response. We will present findings using spatial data visualizations with the aim of informing future disaster preparedness and resilience.
HIST 1230: In U.S. history, racial differences and discrimination have powerfully shaped who benefited from land and farm ownership. How can historians use data to understand the history of race and farming? Students will wrangle county- and state-level data from the U.S. Census of Agriculture from 1840-1912 to create visualizations and apps that allow us to find patterns in the history of race and land, to discover new questions we might not know to ask, and to create tools to better reveal connections between race, land, and farming for a general audience.
STAT 1230: In this course students will dive into the world of data science by focusing on invasive species monitoring data. Early detection is crucial to controlling many invasive species; however, there is a knowledge gap regarding the sampling effort needed to detect the invader early. In this course, we will work with decades of invasive species monitoring data collected across the United States to better understand how environmental variables play a role in the sampling effort required to detect invasive species. Students will gain experience in the entire data science pipeline, but the primary focus will be on data scraping, data visualization, and communication of data-based results to scientists and policymakers.
SOCI 1230: Do sports fans care about climate change? Can sports communication be used to engage audiences on environmental sustainability? In this section of the course, students will use the tools of data science to examine whether interest in sports is associated with climate change knowledge, attitudes and behaviors, as well as other political opinions. Participants will use survey data to produce visualizations and exploratory analyses about the relationship between sports fandom and attitudes about environmental sustainability.
Terms Taught
Requirements
INTD 1230
DataScience Across Disciplines
Course Description
Data Science Across Disciplines
In this course, we will gain exposure to the entire data science pipeline—obtaining and cleaning large and messy data sets, exploring these data and creating engaging visualizations, and communicating insights from the data in a meaningful manner. During morning sessions, we will learn the tools and techniques required to explore new and exciting data sets. During afternoon sessions, students will work in small groups with one of several faculty members on domain-specific research projects in Geography, Political Science, Restorative Justice, or Healthcare. This course will use the R programming language. No prior experience with R is necessary.
INTD 1230 A: Data is a powerful tool for improving health outcomes by making programmatic choices to support justice. In this afternoon section of Data Across the Disciplines, students will be working with Addison County Restorative Justice (ACRJ) on understanding patterns in the occurrence of driving under the influence. ACRJ has over 1,000 cases and would like to better understand their data and come up with ways to access information. We will explore how identity, geography, and support impact outcomes from DUI cases. Using statistical analysis and data visualizations, along with learning about ethical data practices, we will report our findings.
INTD 1230 B: Let’s dive into the minutes and reports of local towns to develop an accessible news and history resource. Could this be a tool for small newspapers to track local news more easily? Can we map this fresh data for a new look across geographies? Do you want to help volunteer town officials make decisions and better wrangle with their town’s history and data? In this course we will develop a focused database of documents produced by several municipal boards and commissions. We will engage in conversation with local officials, researchers, and journalists. This course aims to introduce students to making data from real world documents and the people that make them to generate useful information that is often open but frequently difficult to sift through.
GEOG 1230: In this section, students will use data science tools to explore the ways migration systems in the United States changed during the COVID-19 pandemic. We will draw on data collected from mobile phones recording each phone’s monthly place of residence at the census tract level. The dataset includes monthly observations from January 2019 through December 2021 allowing the analysis to compare migration systems pre-pandemic with those during the pandemic.
MATH/STAT 1230: Students will explore pediatric healthcare data to better understand the risks correlated with various childhood illnesses through an emphasis on the intuition behind statistical and machine learning techniques. We will practice making informed decisions from noisy data and the steps to go from messy data to a final report. Students will become proficient in R and gain an understanding of various statistical techniques.
PSCI 1230: How do candidates for U.S. national office raise money? From whom do they raise it? In this section we will explore these questions using Federal Election Commission data on individual campaign contributions to federal candidates. Our analysis using R will help us identify geographic patterns in the data, as well as variations in funds raised across types of candidates. We will discuss what implications these patterns may have for the health and functioning of democracy in the U.S.
Terms Taught
Requirements
MATH 0106
Math and Board Games
Course Description
Math and Board Games
Have you ever spent minutes agonizing over which move to make in a board game? Out of all the possible options, how could you possibly determine which move is best? Was there even an objectively best decision? In this course, we will explore the mathematics and underlying gameplay structures of several modern board games. In addition to playing these games during class, we’ll use math and logic to assess and quantify the value of a range of possible in-game decisions. Using formal mathematical proofs, papers, and in-class discussions, we’ll analyze the fairness and equity of strategies across a wide variety of games. We’ll finish the course by designing our own board game based on what we’ve learned! (Students who have completed FYSE1216 are not eligible to enroll in MATH 0106.)
Terms Taught
Requirements
MATH 0116
Intro to Statistical Science
Course Description
Introduction to Statistical Science
A practical introduction to statistical methods and the examination of data sets. Computer software will play a central role in analyzing a variety of real data sets from the natural and social sciences. Topics include descriptive statistics, elementary distributions for data, hypothesis tests, confidence intervals, correlation, regression, contingency tables, and analysis of variance. The course has no formal mathematics prerequisite, and is especially suited to students in the physical, social, environmental, and life sciences who seek an applied orientation to data analysis. (Credit is not given for MATH 0116 if the student has taken ECON 0111 (formerly ECON 0210) or PSYC 0201 previously or concurrently.) 3 hrs. lect./1 hr. computer lab.
Terms Taught
Requirements
MATH 0118
Introduction to Data Science
Course Description
Introduction to Data Science
In this course students will gain exposure to the entire data science pipeline: forming a statistical question, collecting and cleaning data sets, performing exploratory data analyses, identifying appropriate statistical techniques, and communicating the results, all the while leaning heavily on open source computational tools, in particular the R statistical software language. We will focus on analyzing real, messy, and large data sets, requiring the use of advanced data manipulation/wrangling and data visualization packages. Students will be required to bring alaptop (owned or college-loaned) to class as many lectures will involve in-class computational activities. (formerly MATH216) 3 hrs lect./disc. (Not open to students who have taken BIOL 1230, ECON 1230, ENVS 1230, FMMC 1230, HARC 1230, JAPN 1230, LNGT 1230, NSCI 1230, MATH 1230, SOCI 1230, LNGT 1230, PSCI 1230, WRPR 1230, or GEOG 1230.)
Terms Taught
Requirements
MATH 0216
Introduction to Data Science
Course Description
Introduction to Data Science
In this course students will gain exposure to the entire data science pipeline: forming a statistical question, collecting and cleaning data sets, performing exploratory data analyses, identifying appropriate statistical techniques, and communicating the results, all the while leaning heavily on open source computational tools, in particular the R statistical software language. We will focus on analyzing real, messy, and large data sets, requiring the use of advanced data manipulation/wrangling and data visualization packages. Students will be required to bring their own laptops as many lectures will involve in-class computational activities. 3 hrs lect./disc.
Terms Taught
Requirements
MATH 0218
Statistical Learning
Course Description
Statistical Learning
This course is an introduction to modern statistical, machine learning, and computational methods to analyze large and complex data sets that arise in a variety of fields, from biology to economics to astrophysics. The theoretical underpinnings of the most important modeling and predictive methods will be covered, including regression, classification, clustering, resampling, and tree-based methods. Student work will involve implementation of these concepts using open-source computational tools. (MATH 0118, or MATH 0216, or BIOL 1230, or ECON 1230, or ENVS 1230, or FMMC 1230, or HARC 1230, or JAPN 1230, or LNGT 1230, or NSCI 1230, or MATH 1230 or SOCI 1230) 3 hrs. lect./disc.
Terms Taught
Requirements
MATH 0311
Statistical Inference
Course Description
Statistical Inference
An introduction to the mathematical methods and applications of statistical inference using both classical methods and modern resampling techniques. Topics will include: permutation tests, parametric and nonparametric problems, estimation, efficiency and the Neyman-Pearsons lemma. Classical tests within the normal theory such as F-test, t-test, and chi-square test will also be considered. Methods of linear least squares are used for the study of analysis of variance and regression. There will be some emphasis on applications to other disciplines. This course is taught using R. (MATH 0310) 3 hrs. lect./disc.
Terms Taught
Requirements
MATH 0500
Upcoming
Advanced Study
Course Description
Advanced Study
Individual study for qualified students in more advanced topics in algebra, number theory, real or complex analysis, topology. Particularly suited for those who enter with advanced standing. (Approval required) 3 hrs. lect./disc.
Terms Taught
MATH 0711
Statistics Capstone Seminar
Course Description
Statistics Capstone Seminar
In this course we will work with community partners to solve real-world problems using modern statistical and data science techniques. Students will work in small groups to translate research questions into actionable analysis and visualizations. Students will select a project of interest from a subset of community partners, maintain contact and collaboration with the community partner, and present their findings in a final symposium. (MATH 0218, MATH 0311, or by approval) 3 hrs. sem.
Terms Taught
Requirements
MBBC 0700
Upcoming
Senior Independent Research
Course Description
Senior Independent Research
Seniors conducting independent research in Molecular Biology and Biochemistry under the guidance of a faculty mentor should register for MBBC 0700 unless they are completing a thesis project (in which case they should register for MBBC 0701). Additional requirements include attendance at all MBBC-sponsored seminars and seminars sponsored by the faculty mentor’s department, and participation in any scheduled meetings and disciplinary sub-groups and lab groups. (Approval required).
Terms Taught
MBBC 0701
Upcoming
Senior Thesis
Course Description
Senior Thesis
This course is for seniors completing independent thesis research in Molecular Biology and Biochemistry that was initiated in BIOL 0500, CHEM 0400, MBBC 0500, or MBBC 0700. Students will attend weekly meetings with their designated research group and engage in one-on-one meetings with their research mentor to foster understanding in their specialized research area. Students will also practice the stylistic and technical aspects of scientific writing needed to write their thesis. (BIOL 0500, CHEM 0400, MBBC 0500, MBBC 0700) (Approval required).
Terms Taught
NSCI 1230
DataScience Across Disciplines
Course Description
Data Science Across Disciplines
In this course, we will gain exposure to the entire data science pipeline—obtaining and cleaning large and messy data sets, exploring these data and creating engaging visualizations, and communicating insights from the data in a meaningful manner. During morning sessions, we will learn the tools and techniques required to explore new and exciting data sets. During afternoon sessions, students will work in small groups with one of several faculty members on domain-specific research projects in Sociology, Neuroscience, Animation, Art History, or Environmental Science. This course will utilize the R programming language. No prior experience with R is necessary.
ENVS: Students will engage in research within environmental health science—the study of reciprocal relationships between human health and the environment. High-quality data and the skills to make sense of these data are key to studying environmental health across diverse spatial scales, from individual cells through human populations. In this course, we will explore common types of data and analytical tools used to answer environmental health questions and inform policy.
FMMC: Students will explore how to make a series of consequential decisions about how to present data and how to make it clear, impactful, emotional or compelling. In this hands-on course we will use a wide range of new and old art making materials to craft artistic visual representations of data that educate, entertain, and persuade an audience with the fundamentals of data science as our starting point.
NSCI/MATH: Students will use the tools of data science to explore quantitative approaches to understanding and visualizing neural data. The types of neural data that we will study consists of electrical activity (voltage and/or spike trains) measured from individual neurons and can be used to understand how neurons respond to and process different stimuli (e.g., visual or auditory cues). Specifically, we will use this neural data from several regions of the brain to make predictions about neuron connectivity and information flow within and across brain regions.
SOCI: Students will use the tools of data science to examine how experiences in college are associated with social and economic mobility after college. Participants will combine sources of "big data" with survey research to produce visualizations and exploratory analyses that consider the importance of higher education for shaping life chances.
HARC: Students will use the tools of data science to create interactive visualizations of the Dutch textile trade in the early eighteenth century. These visualizations will enable users to make connections between global trade patterns and representations of textiles in paintings, prints, and drawings.
Terms Taught
Requirements
PSCI 1230
DataScience Across Disciplines
Course Description
Data Science Across Disciplines
In this course, we will gain exposure to the entire data science pipeline—obtaining and cleaning large and messy data sets, exploring these data and creating engaging visualizations, and communicating insights from the data in a meaningful manner. During morning sessions, we will learn the tools and techniques required to explore new and exciting data sets. During afternoon sessions, students will work in small groups with one of several faculty members on domain-specific research projects in Geography, Political Science, Restorative Justice, or Healthcare. This course will use the R programming language. No prior experience with R is necessary.
PSCI 1230: How do candidates for U.S. national office raise money? From whom do they raise it? In this section we will explore these questions using Federal Election Commission data on individual campaign contributions to federal candidates. Our analysis using R will help us identify geographic patterns in the data, as well as variations in funds raised across types of candidates. We will discuss what implications these patterns may have for the health and functioning of democracy in the U.S.
INTD 1230A: Data is a powerful tool for improving health outcomes by making programmatic choices to support justice. In this afternoon section of Data Across the Disciplines, students will be working with Addison County Restorative Justice (ACRJ) on understanding patterns in the occurrence of driving under the influence. ACRJ has over 1,000 cases and would like to better understand their data and come up with ways to access information. We will explore how identity, geography, and support impact outcomes from DUI cases. Using statistical analysis and data visualizations, along with learning about ethical data practices, we will report our findings.
INTD 1230B: Let’s dive into the minutes and reports of local towns to develop an accessible news and history resource. Could this be a tool for small newspapers to track local news more easily? Can we map this fresh data for a new look across geographies? Do you want to help volunteer town officials make decisions and better wrangle with their town’s history and data?
In this course we will develop a focused database of documents produced by several municipal boards and commissions. We will engage in conversation with local officials, researchers, and journalists. This course aims to introduce students to making data from real world documents and the people that make them to generate useful information that is often open but frequently difficult to sift through.
GEOG 1230: In this section, students will use data science tools to explore the ways migration systems in the United States changed during the COVID-19 pandemic. We will draw on data collected from mobile phones recording each phone’s monthly place of residence at the census tract level. The dataset includes monthly observations from January 2019 through December 2021 allowing the analysis to compare migration systems pre-pandemic with those during the pandemic.
MATH/STAT 1230: Students will explore pediatric healthcare data to better understand the risks correlated with various childhood illnesses through an emphasis on the intuition behind statistical and machine learning techniques. We will practice making informed decisions from noisy data and the steps to go from messy data to a final report. Students will become proficient in R and gain an understanding of various statistical techniques.
Terms Taught
Requirements
SOCI 1230
DataScience Across Disciplines
Course Description
Data Science Across Disciplines
In this course, we will gain exposure to the entire data science pipeline—obtaining and cleaning, large and messy data sets, exploring these data and creating engaging visualizations, and communicating insights from the data in a meaningful manner. During morning sessions, we will learn the tools and techniques required to explore new and exciting data sets. During afternoon sessions, students will work in small groups with one of several faculty members on domain-specific research projects in Biology, Geography, History, Mathematics/Statistics and Sociology. This course will use the R programming language. No prior experience with R is necessary.
BIOL 1230: Students enrolled in Professor Casey’s (Biology) afternoon section will use the tools of data science to investigate the drivers of tick abundance and tick-borne disease risk. To do this students will draw from a nation-wide ecological database.
GEOG 1230: In this section, we will investigate human vulnerability to natural hazards in the United States using location-based text data about hurricane and flood disasters from social media. We will analyze data qualitatively, temporally, and spatially to gain insights into the human experience of previous disasters and disaster response. We will present findings using spatial data visualizations with the aim of informing future disaster preparedness and resilience.
HIST 1230: In U.S. history, racial differences and discrimination have powerfully shaped who benefited from land and farm ownership. How can historians use data to understand the history of race and farming? Students will wrangle county- and state-level data from the U.S. Census of Agriculture from 1840-1912 to create visualizations and apps that allow us to find patterns in the history of race and land, to discover new questions we might not know to ask, and to create tools to better reveal connections between race, land, and farming for a general audience.
STAT 1230: In this course students will dive into the world of data science by focusing on invasive species monitoring data. Early detection is crucial to controlling many invasive species; however, there is a knowledge gap regarding the sampling effort needed to detect the invader early. In this course, we will work with decades of invasive species monitoring data collected across the United States to better understand how environmental variables play a role in the sampling effort required to detect invasive species. Students will gain experience in the entire data science pipeline, but the primary focus will be on data scraping, data visualization, and communication of data-based results to scientists and policymakers.
SOCI 1230: Do sports fans care about climate change? Can sports communication be used to engage audiences on environmental sustainability? In this section of the course, students will use the tools of data science to examine whether interest in sports is associated with climate change knowledge, attitudes and behaviors, as well as other political opinions. Participants will use survey data to produce visualizations and exploratory analyses about the relationship between sports fandom and attitudes about environmental sustainability.
Terms Taught
Requirements
STAT 0201
Intro to Stat and Data Sci
Course Description
Introduction to Statistical and Data Sciences
An introduction to statistical methods and the examination of data sets for students with a background in calculus. Topics include descriptive statistics, elementary distributions for data, hypothesis tests, confidence intervals, and regression. Students develop skills in data cleaning, wrangling, visualization, and model fitting using the Statistical Software R. Emphasis will be placed on reproducibility. (MATH 0121 or APAB 4 or APBC 3, or by waiver) (Not open to students who have taken MATH 0116, MATH 0118, ECON 0111 (formerly ECON 0210), PSYC 0201, STAT 0116, STAT 0118, BIOL 1230, ECON 1230, ENVS 1230, FMMC 1230, HARC 1230, JAPN 1230, LNGT 1230, NSCI 1230, MATH 1230, SOCI 1230, LNGT 1230, PSCI 1230, WRPR 1230, or GEOG 1230.)
Terms Taught
Requirements
STAT 0218
Statistical Learning
Course Description
Statistical Learning (formerly MATH 0218)
This course is an introduction to modern statistical, machine learning, and computational methods to analyze large and complex data sets that arise in a variety of fields, from biology to economics to astrophysics. The theoretical underpinnings of the most important modeling and predictive methods will be covered, including regression, classification, clustering, resampling, and tree-based methods. Student work will involve implementation of these concepts using open-source computational tools. (MATH 0118 or STAT 0118 or STAT 0201 or MATH 0216 or BIOL 1230 or ECON 1230 or ENVS 1230, or FMMC 1230 or HARC 1230 or JAPN 1230 or LNGT 1230 or NSCI 1230 or MATH 1230 or SOCI 1230or WRPR 1230 or GEOG 1230) 3 hrs. lect./disc.
Terms Taught
Requirements
STAT 0350
Upcoming
Randomness & Strategy in Games
Course Description
Randomness and Strategy in Video Games
Colloquially, randomness is the lack of predictability and pattern. In statistics, randomness describes events whose outcomes are unknown but whose behavior is characterized by probability distributions. In this course, we will explore a variety of implementations of both input and output randomness in modern video games and assess how they inform strategy and affect the user experience. Do random events yield a variety of strategies, a dominant one, or no strategy at all? How does randomness relate to user experience? We will explore the answers to these questions and more in an exploration of the intersection of statistics and video game design. (MATH/STAT 0310)
Terms Taught
Requirements
STAT 0500
Upcoming
Advanced Study
Course Description
Independent Study
Individual study for qualified students in more advanced topics in statistics. Particularly suited for those who enter with advanced standing. (Approval required) 3 hrs. lect./disc.
Terms Taught
STAT 0711
Upcoming
Statistical Consulting
Course Description
Statistical Consulting
In this course we will work with community partners to solve real-world problems using modern statistical and data science techniques. Students will work in small groups to translate research questions into actionable analysis and visualizations. Students will select a project of interest from a subset of community partners, maintain contact and collaboration with the community partner, and present their findings in a final symposium.
(MATH 0218 or STAT 0218 or MATH 0311 or STAT 0311 or by approval) 3 hrs. sem.
Terms Taught
Requirements
STAT 1230
DataScience Across Disciplines
Course Description
Data Science Across Disciplines
In this course, we will gain exposure to the entire data science pipeline—obtaining and cleaning, large and messy data sets, exploring these data and creating engaging visualizations, and communicating insights from the data in a meaningful manner. During morning sessions, we will learn the tools and techniques required to explore new and exciting data sets. During afternoon sessions, students will work in small groups with one of several faculty members on domain-specific research projects in Biology, Geography, History, Mathematics/Statistics and Sociology. This course will use the R programming language. No prior experience with R is necessary.
BIOL 1230: Students enrolled in Professor Casey’s afternoon section will use the tools of data science to investigate the drivers of tick abundance and tick-borne disease risk. To do this students will draw from a nation-wide ecological database.
GEOG 1230: In this section, we will investigate human vulnerability to natural hazards in the United States using location-based text data about hurricane and flood disasters from social media. We will analyze data qualitatively, temporally, and spatially to gain insights into the human experience of previous disasters and disaster response. We will present findings using spatial data visualizations with the aim of informing future disaster preparedness and resilience.
HIST 1230: In U.S. history, racial differences and discrimination have powerfully shaped who benefited from land and farm ownership. How can historians use data to understand the history of race and farming? Students will wrangle county- and state-level data from the U.S. Census of Agriculture from 1840-1912 to create visualizations and apps that allow us to find patterns in the history of race and land, to discover new questions we might not know to ask, and to create tools to better reveal connections between race, land, and farming for a general audience.
STAT 1230: In this course students will dive into the world of data science by focusing on invasive species monitoring data. Early detection is crucial to controlling many invasive species; however, there is a knowledge gap regarding the sampling effort needed to detect the invader early. In this course, we will work with decades of invasive species monitoring data collected across the United States to better understand how environmental variables play a role in the sampling effort required to detect invasive species. Students will gain experience in the entire data science pipeline, but the primary focus will be on data scraping, data visualization, and communication of data-based results to scientists and policymakers.
SOCI 1230: Do sports fans care about climate change? Can sports communication be used to engage audiences on environmental sustainability? In this section of the course, students will use the tools of data science to examine whether interest in sports is associated with climate change knowledge, attitudes and behaviors, as well as other political opinions. Participants will use survey data to produce visualizations and exploratory analyses about the relationship between sports fandom and attitudes about environmental sustainability.
Terms Taught
Requirements